When you study a group of individuals, gathering data from each member can be challenging. It’s nearly impossible to get data from every person individually while researching a large group of people. Instead, you pick a sample. The sample is the population that will take part in the research study.
Choosing the most appropriate sampling method for your research is essential. Cluster and stratified sampling are distinct probability sampling procedures for creating and analyzing samples. This article will explain the definition of Cluster sampling and Stratified sampling, Cluster sampling vs. Stratified sampling examples, and differences between cluster vs. stratified sampling.
Let's start with the definitions
Cluster sampling and stratified sampling may appear comparable, but the groups formed in the latter method are heterogeneous, meaning each cluster has different individual characteristics. Stratified sampling, conversely, results in homogeneous groupings as the units are similar.
Cluster sampling
Cluster sampling is a standard probability sampling technique for studying sizable populations dispersed across a large region. Thus, using a straightforward or systematic random sampling strategy, researchers select random groups for data collection and analysis.
Cluster sampling uses one-stage, two-stage, and multiple-stage sampling procedures, depending on how many stages are needed to obtain the required sample. This sampling technique is incredibly cost-effective because it is easy to use and requires little effort to create samples. Here are the three main cluster sampling types:
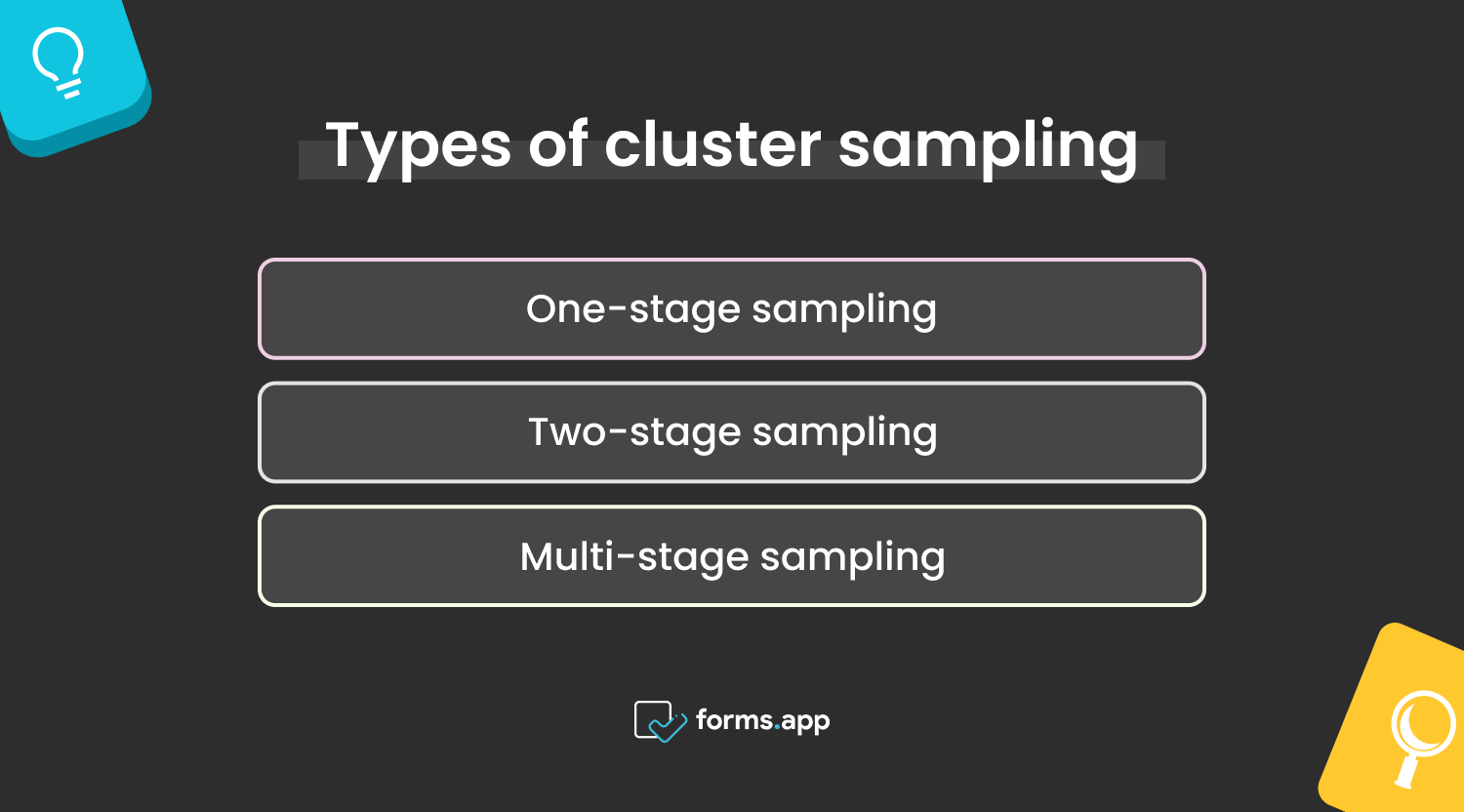
Cluster sampling types
- One-stage sampling: One-stage sampling, or single-stage cluster sampling, is a technique in which every element from the selected clusters is included in the sample group. Thus, all individuals within the chosen clusters can participate in the scientific analysis using one-stage sampling.
- Two-stage sampling: The researcher uses a two-stage sampling method to choose the study sample in two stages. Subgroups randomly select a sample at the first stage of sampling, known as single-stage sampling. Next, the sample size is lowered by choosing a limited subset of study participants from the selected cluster.
- Multi-stage sampling: Using multi-stage sampling, the researcher can select a subset of the target population for further examination. After selecting the two-stage sample, the researcher uses established criteria to choose the study sample.
The sampling technique is applied in market research, while geographic locations or area cluster sampling. A vast geographic area may incur more costs when compared to surveys distributed to cluster sampling divided by region. You can examine the cluster sampling example below to understand better:
Example: A researcher wants to conduct a study to evaluate the performance of third-year teaching students across Canada. It is not feasible to carry out research that includes every university student. Instead, the researcher can use cluster sampling to create a single cluster comprising all universities in a city.
After that, these clusters are found by either systematic or simple random sampling. The clusters are chosen randomly for the research study to determine the population of third-year students in Canada. Third-grade students in these selected groups can then be chosen to perform the research study through simple or systematic sampling.
Stratified sampling
Stratified sampling, or random quota sampling, is a probability sampling technique in which a sizable population is separated into distinct, homogeneous strata. Then, individuals from these strata are randomly chosen to create a sample. This technique chooses participants randomly from each stratum after dividing the population into smaller groups according to specific criteria.
Since each sample will have unique components, every member of the population will have an equal chance to be included in these samples. This sampling method may be used to separate people based on various criteria, such as socioeconomic situation, age, gender, and nationality. Stratification can be proportional or disproportionate.
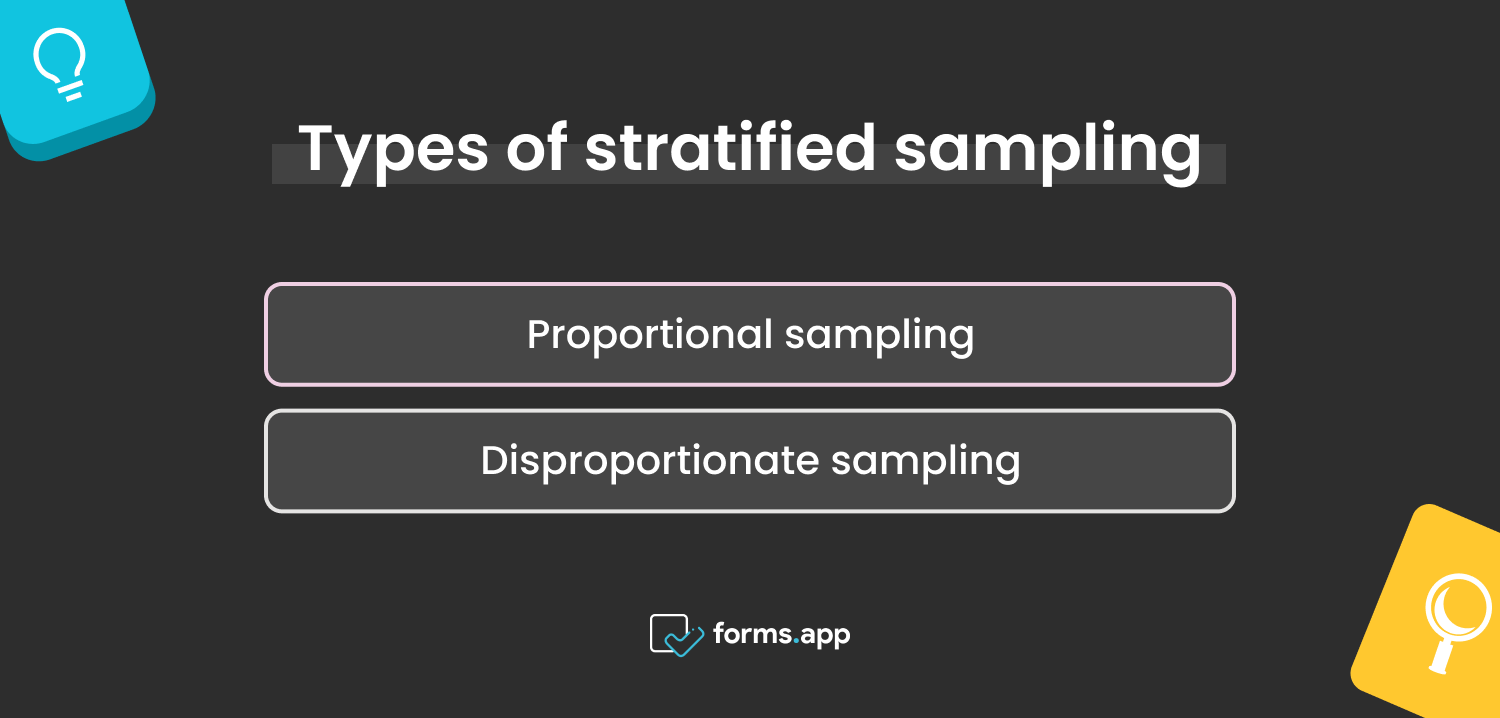
Stratified sampling types
- Proportional sampling: In a proportional stratified sampling technique, the sample size in each stratum is inversely associated with the population size. This form of stratified random sampling usually yields a more accurate measurement as it more closely represents the whole population.
- Disproportionate sampling: In a disproportionally stratified sample, the size of each stratum differs from its size in the population. The effectiveness of the sampling procedure will depend on how precisely the researcher divides fractions. If the subsets assigned are ambiguous, the findings may be distorted by over or underrepresented strata.
Example: Suppose a private healthcare institution wants to conduct a hospital services satisfaction survey among its diverse patient base, which consists of three age groups: 18-35, 36-55, and over 55. The health organization uses stratified sampling to ensure survey results represent age groups.
First, the healthcare organization divides its customer base into ages 18-35, 36-55, and over 55. A random sample of customers from each age group is then selected to participate in the survey, ensuring that each age group is adequately represented.
Cluster sampling vs. Stratified sampling
A stratified sampling includes splitting the population into strata or subgroups depending on gender, age, income, and education level. In contrast, cluster sampling entails separating the population into groupings or clusters, such as organizations, institutions, communities, or associations. Here are some specific differences between stratified vs cluster sampling:
- Variability: Using stratified sampling leads to increased variability across layers and decreased variability within the strata. When using cluster sampling, there is less variability across clusters and more variability within clusters.
- Objective: While cluster sampling focuses on improving the speed and convenience of data collection, stratified sampling seeks to decrease variability and enhance the sample’s representativeness.
- Sampling strategy: Whereas cluster sampling divides the population into naturally occurring clusters, stratified sampling divides the population into specific subgroups or strata.
- Sample size: Stratified sampling often necessitates a larger sample size, unlike cluster sampling, which can achieve comparable accuracy levels with a smaller sample size.
- Cost: Stratified sampling may result in higher expenditures due to the bigger sample size. However, the expenses may be cheaper because the sample size is smaller, and the sampling procedure is more straightforward with cluster sampling.
- Complexity: Stratified sampling is typically more intricate and time-consuming because it requires creating strata and multi-level data analysis. Cluster sampling is comparatively more superficial and simpler.
- Data analysis: Stratified analysis, in which data from each stratum is examined independently, is required for stratified sampling. Cluster-based analysis, in which data from every cluster is reviewed as a whole, is necessary for cluster sampling.
Conclusion
In conclusion, stratified sampling chooses participants randomly from each stratum after the population has been divided into subgroups according to specific criteria. On the other hand, the cluster sampling process groups the population into clusters and then chooses complete clusters randomly to include in the sample.
Both cluster and stratified sampling have core advantages and disadvantages. You can choose the best sampling method for your population size and research type. This article has examined the definition of cluster and stratified sampling methods, the advantages of cluster sampling and stratified sampling, and their differences. Learn every detail about stratified and cluster sampling methods today!
Sena is a content writer at forms.app. She likes to read and write articles on different topics. Sena also likes to learn about different cultures and travel. She likes to study and learn different languages. Her specialty is linguistics, surveys, survey questions, and sampling methods.